Advancements in Digital Biomarkers & Medical Diagnostics
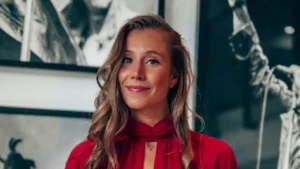
Among the universal benefits that AI technology offers, today’s startup organizations within the diagnostics field are especially likely to capitalize on its various innovations, according to Talia Grace Haller, a consultant who specializes in AI strategizing for clients in the fields of life sciences and healthcare.
“Given their smaller size, dynamic infrastructures, nimble operational frameworks, and innovation-friendly environments, startups are uniquely positioned to harness AI both operationally and within their product designs to enhance efficiency and product quality, as well as to drive that innovation,” Haller told MD+DI.
From an operational standpoint, AI has the potential to empower startups by providing more efficient opportunities to utilize their limited resources while pivoting strategically as needed in ways that offer significant advantages and incentives to attract leading industry talent, she said. One such example involves what Haller describes as “the revolutionary intersection of biomarker discovery and AI” that is setting new standards for diagnostic technologies.
During the upcoming MEDevice conference in Boston, MA, Sept. 25-26, Haller will present the session, Innovating Diagnostics: AI-Driven Biomarker Discovery for Medical Device Start-ups to educate entrepreneurs and innovators at the forefront of medtech development on the latest advancements in biomarkers and their significance in the evolution of early disease detection, precise prognosis, and individualized treatment modalities.
Haller recently participated in a Q&A with MD+DI to discuss some of the influential concept’s AI holds today, including the progression and ethical challenges of AI markup language (AIML) in bringing digital diagnostics full circle when considering how information is interpreted and communicated to patients today.
What do you see as the biggest impact in the diagnostic field today given more precise diagnoses with the help of AIML?
Haller: The impacts of AIML in diagnostics cannot be overemphasized. It will be huge, profound, and life-changing beyond imagination. First and foremost, people who want it will increasingly gain control of their health. But it will be an active choice, especially initially.
For those with access to AI-driven diagnostics and integrated multiomics, the era of not knowing what’s wrong will soon be over. The days of late-stage cancer diagnoses will be a thing of the past. The trial-and-error approach to therapy selection will become obsolete. And the practice of once-per-year doctor appointments will be replaced by continuous, proactive health monitoring.
Our biomarkers are continuously monitored in real-time. Individuals can live their lives with AI running in the background. When something abnormal arises, AI will flag it and recommend the best course of action, such as additional diagnostic testing or consulting with a medical professional.
A continuous liquid biopsy monitor of some sort will detect the earliest signs of cancer, which can then be taken care of with the optimal precision therapy. Gene editing and other advanced tools and medications can be leveraged to treat health conditions that arise in an extremely efficient and timely manner, which will allow people to live longer and in better health if they make their health a priority. But it’s important to note that having control over one’s health is not the same as achieving perfect health. What AI truly offers individuals are choices, empowering them to evaluate and decide on the best path to live their lives to the fullest.
The term innovation has become ubiquitous as it relates to advanced technology. What do you think is most innovative about the use of AIML for diagnostic purposes?
Haller: The phrase innovative technology refers to a constantly moving target, as innovation inherently looks for new advancements. At one point, vaccines, anesthesia, and antibiotics were all extremely innovative. We’ve arrived at a new point in history where the amount of data that can be generated about one patient is simply too much for a single individual mind to ever comprehend, let alone in one [patient] appointment time slot, which means we must rely on AI to help us extract insights from the growing pool of data. In 2024, there are three things that stand out as very innovative when it comes to the use of AIML for diagnostic purposes:
-
Integration of multiomics data: A foundational innovation that enables other advancements in AI for diagnostics. By combining various biological datasets, such as genomics, transcriptomics, proteomics, metabolomics, and exposomics, AI can integrate diagnostic device data with a patient’s multiomics profile. This provides an unprecedented view of a patient’s overall health and how various biomarkers move in relation to one another.
-
Predictive analytics for early disease detection and optimized care recommendations: AI can analyze large datasets from electronic health records, genetic data, and other sources to predict the onset of diseases before symptoms appear. This enables early intervention and preventive care, which can significantly improve patient outcomes and reduce healthcare costs. By analyzing data from previous cases, clinical trials, and medical literature, AI systems can suggest the most effective treatments and predict potential side effects, leading to more effective and safer therapies.
-
Digital twin personalization: For personalized simulations of disease progression, treatment responses, and overall health outcomes. By comparing a digital twin to a database of other twins, healthcare providers can generate personalized reference ranges, predict disease onset, and tailor treatments to individual needs.
How do you describe what the intersection of biomarker discovery and AI means as it relates to how this technology has evolved to where we are today for diagnostic purposes?
Haller: This refers to the synergistic use of AI technologies to enhance the identification and validation of biomarkers for diagnostic purposes. Historically, biomarker discovery relied on manual, labor-intensive processes, such as laboratory experiments and clinical trials. These methods were time-consuming, expensive, and often limited in scope and scalability. The advent of high-throughput technologies, such as next-generation sequencing and mass spectrometry, has enabled the generation of vast amounts of biological data, including genomics, proteomics, and metabolomics. While these technologies have increased the potential for biomarker discovery, the volume and complexity of data generated pose significant analytical challenges. AI can integrate and harmonize diverse types of biological data, enabling a comprehensive analysis of biological systems. Advanced machine learning algorithms, including deep learning, excel at recognizing complex patterns and associations within large datasets, which traditional methods might miss. AI can build predictive models to identify potential biomarkers by correlating biological data with clinical outcomes, which can then be validated and refined using additional datasets. AI accelerates the biomarker discovery process by rapidly analyzing large datasets, automating repetitive tasks, and providing real-time insights, significantly reducing the time and cost involved.
Have there been any ethical concerns related to the use of AIML in this capacity?
Haller: There are numerous ethical concerns and even philosophical questions that arise with the use of AIML in diagnostics. Ethical concerns include privacy and data security, bias and fairness, transparency, and accountability. The vast amount of personal health data required for AIML systems to function effectively poses significant privacy risks. Ensuring that data is securely stored and managed, and that patient confidentiality is maintained, is paramount. The risk of data breaches and unauthorized access to sensitive health information is also a critical concern. Protecting against such incidents is crucial to maintain patient trust and to comply with legal standards.
Algorithmic bias occurs when AIML systems inadvertently perpetuate or even exacerbate existing biases in healthcare. If training data is biased, diagnostic recommendations will be biased, potentially leading to unequal treatment across different demographic groups. Ensuring that all patients, regardless of socioeconomic status, have equal access to the benefits of AI-driven diagnostics is also a major ethical challenge.
As it pertains to transparency and accountability, many AI models, particularly deep learning algorithms, operate as black boxes, where decision-making processes are not transparent. This lack of transparency can make it difficult to understand how diagnostic decisions are made and how to hold the system accountable for errors.
What are the ethical obligations that providers have today to inform patients about the use and results related to AIML methods?
Haller: There is a growing demand for AI systems to provide explanations for their diagnoses in a way that clinicians and patients can understand, ensuring that the decision-making process is transparent and trustworthy. If a diagnostic device or test reveals materially impactful information about a patient’s health, companies face ethical and legal questions about their obligation to inform the patient. This involves a process where a doctor should ideally be involved to provide context and support.
If the information also pertains to genetically related family members, others in a similar geographic region, or the same digital twin cohort, the ethical dilemma extends further. Should the company inform others who did not directly seek their care? If inclined to inform, what would this process entail, and how would privacy be safeguarded? What happens if a diagnostic device provides materially impactful negative news that turns out to be incorrect? If a patient takes action based on this incorrect information, it can lead to serious consequences. Determining accountability between the device manufacturer, the AI developers, and the healthcare providers is complex and currently a gray area in legal terms. Clear guidelines and protocols must be established for informing patients and potentially impacted individuals about materially impactful health information, ensuring accuracy, and delineating legal accountability. As we navigate these complexities, it is crucial to develop ethical guidelines and regulatory frameworks that ensure the responsible use of AI in healthcare, ultimately enhancing patient outcomes and maintaining public trust.
What are the types of philosophical questions that can arise with the use of AIML in diagnostics?
Haller: At its heart, AI in diagnostics aims to optimize each individual’s health. But this leads to a deeper question: What does optimal health truly mean? Historically, our focus has been on keeping people alive and disease-free, or, at the very least, minimizing the impact of diseases. However, we are now entering an era where we have unprecedented control over health. It’s not just about keeping people visibly disease-free and alive; we will have the ability to understand health at such a deep level that we can optimize it in ways previously unimaginable. As we optimize health, we will begin to discover the true limits of human longevity.
This raises profound questions. If we optimize our health to the fullest extent, how long can we live? With advancements such as organ replacements, gene editing, and precision medicine, is it possible to significantly extend the human lifespan, or even achieve some form of immortality?
These questions are not just scientific but deeply philosophical. They challenge our current understanding of life and death, the nature of human existence, and the ethical boundaries of medical intervention. As we push the limits of what is possible with AIML, we must carefully consider the implications of these advancements, ensuring that they are used in ways that are ethical and aligned with our values as a society.
Does the recent decision on laboratory developed tests (LDTs) by FDA impact this discussion at all?
Haller: Indeed, it does. The FDA’s new regulations on LDTs mark a significant shift in test management, emphasizing patient safety and device efficacy. For AIML diagnostics, this means adhering to stricter guidelines, which could increase the costs and time needed to bring new AIML diagnostics to market. However, it could also enhance the reliability and acceptance of these technologies in clinical settings. Despite the challenges, particularly regarding compliance and ethical considerations, the potential benefits in improving patient care and diagnostic accuracy make this a critical area of focus for the future of healthcare.
Article Source: MDDI